How to convert percent to decimal. In order to convert percent to decimal number, the percentage should be divided by 100: 1% = 1 / 100 = 0.01. 5% = 5/100 = 0.05. We use cookies on Kaggle to deliver our services, analyze web traffic, and improve your experience on the site. By using Kaggle, you agree to our use of cookies. Markdown Mania: Sold by Markdown Mania: Details. Ships from Markdown Mania. 360° VIEW IMAGES Got2b Color Heads Colored Spiking Styling Glue Metallic Purple 50 Ml (2 Pack) Brand: N'iceshop. 3.5 out of 5 stars 2 ratings. Price: $21.35 ($21.35 / Item) & FREE Shipping: Got2b Color Heads Colored Spiking Styling Glue Metallic Purple 50 Ml (2.
Databricks released this image in January 2019.
Databricks Runtime 5.2 ML provides a ready-to-go environment for machine learning and data science based on Databricks Runtime 5.2 (Unsupported).Databricks Runtime for ML contains many popular machine learning libraries, including TensorFlow, PyTorch, Keras, and XGBoost. It also supports distributed TensorFlow training using Horovod.
For more information, including instructions for creating a Databricks Runtime ML cluster, see Databricks Runtime for Machine Learning.
New features
Databricks Runtime 5.2 ML is built on top of Databricks Runtime 5.2. For information on what's new in Databricks Runtime 5.2, see the Databricks Runtime 5.2 (Unsupported) release notes. In addition to library updates, Databricks Runtime 5.2 ML introduces the following new features:
- GraphFrames now supports the Pregel API (Python) with Databricks's performance optimizations.
- HorovodRunner adds:
- On a GPU cluster, training processes are mapped to GPUs instead of worker nodes to simplify the support of multi-GPU instance types. This built-in support allows you to distribute to all of the GPUs on a multi-GPU machine without custom code.
HorovodRunner.run()
now returns the return value from the first training process.
Note
Databricks Runtime ML releases pick up all maintenance updates to the base Databricks Runtime release. For a list of all maintenance updates, see Databricks runtime maintenance updates.
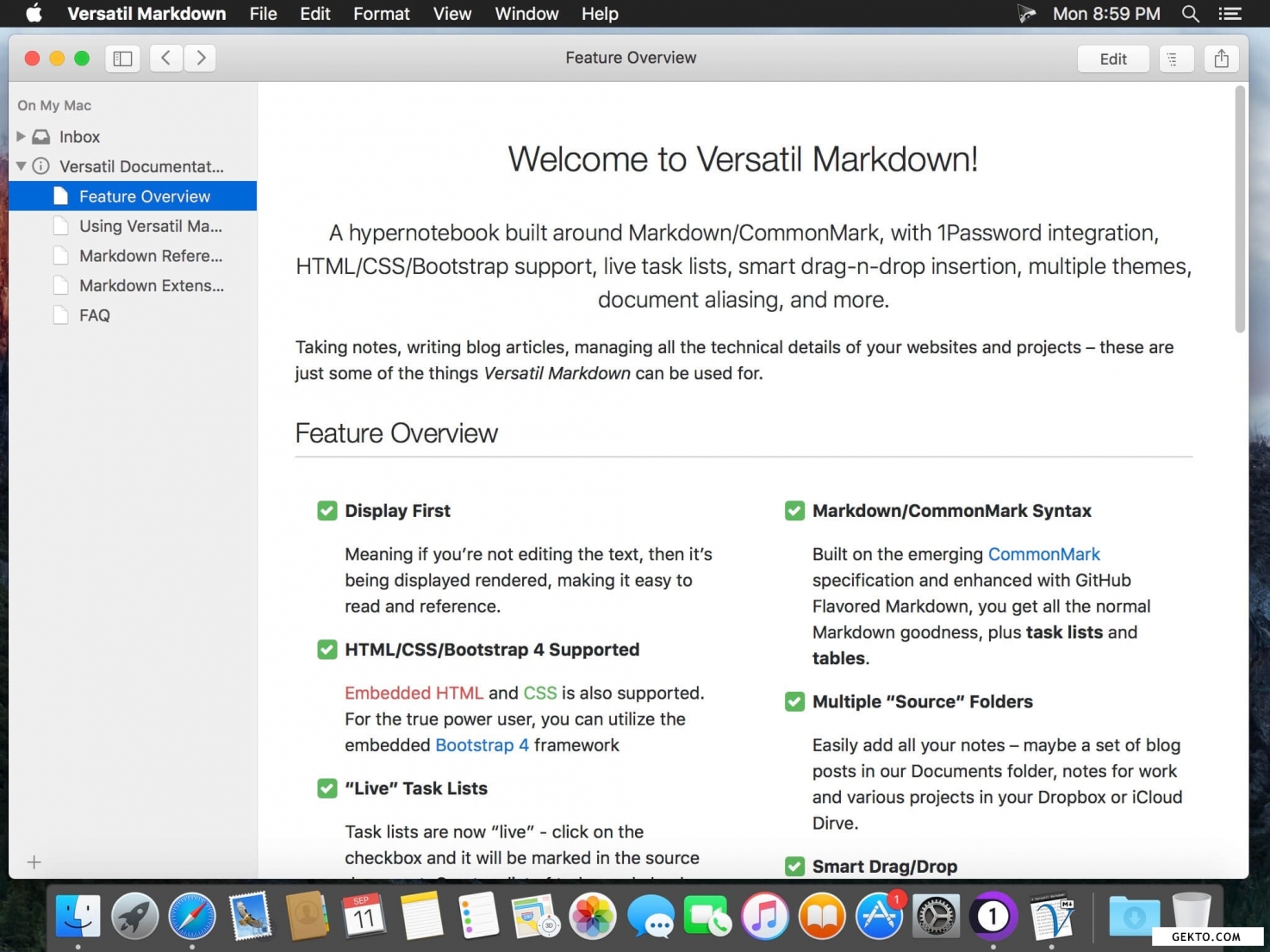
System environment
The system environment in Databricks Runtime 5.2 ML differs from Databricks Runtime 5.2 as follows:
- Python: 2.7.15 for Python 2 clusters and 3.6.5 for Python 3 clusters.
- DBUtils: Databricks Runtime 5.2 ML does not contain Library utilities.
- For GPU clusters, the following NVIDIA GPU libraries:
- Tesla driver 396.44
- CUDA 9.2
- CUDNN 7.2.1
Libraries
Versatil Markdown 2 0 50 Ml =
The following sections list the libraries included in Databricks Runtime 5.2 ML that differ from those included in Databricks Runtime 5.2.
Python libraries
Databricks Runtime 5.2 ML uses Conda for Python package management. As a result, there are major differences in pre-installed Python libraries compared to Databricks Runtime. The following is a full list of provided Python packages and versions installed using Conda package manager.
Library | Version | Library | Version | Library | Version |
---|---|---|---|---|---|
absl-py | 0.6.1 | argparse | 1.4.0 | asn1crypto | 0.24.0 |
astor | 0.7.1 | backports-abc | 0.5 | backports.functools-lru-cache | 1.5 |
backports.weakref | 1.0.post1 | bcrypt | 3.1.5 | bleach | 2.1.3 |
boto | 2.48.0 | boto3 | 1.7.62 | botocore | 1.10.62 |
certifi | 2018.04.16 | cffi | 1.11.5 | chardet | 3.0.4 |
cloudpickle | 0.5.3 | colorama | 0.3.9 | configparser | 3.5.0 |
cryptography | 2.2.2 | cycler | 0.10.0 | Cython | 0.28.2 |
decorator | 4.3.0 | docutils | 0.14 | entrypoints | 0.2.3 |
enum34 | 1.1.6 | et-xmlfile | 1.0.1 | funcsigs | 1.0.2 |
functools32 | 3.2.3-2 | fusepy | 2.0.4 | futures | 3.2.0 |
gast | 0.2.0 | grpcio | 1.12.1 | h5py | 2.8.0 |
horovod | 0.15.2 | html5lib | 1.0.1 | idna | 2.6 |
ipaddress | 1.0.22 | ipython | 5.7.0 | ipython_genutils | 0.2.0 |
jdcal | 1.4 | Jinja2 | 2.10 | jmespath | 0.9.3 |
jsonschema | 2.6.0 | jupyter-client | 5.2.3 | jupyter-core | 4.4.0 |
Keras | 2.2.4 | Keras-Applications | 1.0.6 | Keras-Preprocessing | 1.0.5 |
kiwisolver | 1.0.1 | linecache2 | 1.0.0 | llvmlite | 0.23.1 |
lxml | 4.2.1 | Markdown | 3.0.1 | MarkupSafe | 1.0 |
matplotlib | 2.2.2 | mistune | 0.8.3 | mleap | 0.8.1 |
mock | 2.0.0 | msgpack | 0.5.6 | nbconvert | 5.3.1 |
nbformat | 4.4.0 | nose | 1.3.7 | nose-exclude | 0.5.0 |
numba | 0.38.0+0.g2a2b772fc.dirty | numpy | 1.14.3 | olefile | 0.45.1 |
openpyxl | 2.5.3 | pandas | 0.23.0 | pandocfilters | 1.4.2 |
paramiko | 2.4.1 | pathlib2 | 2.3.2 | patsy | 0.5.0 |
pbr | 5.1.1 | pexpect | 4.5.0 | pickleshare | 0.7.4 |
Pillow | 5.1.0 | pip | 10.0.1 | ply | 3.11 |
prompt-toolkit | 1.0.15 | protobuf | 3.6.1 | psycopg2 | 2.7.5 |
ptyprocess | 0.5.2 | pyarrow | 0.8.0 | pyasn1 | 0.4.4 |
pycparser | 2.18 | Pygments | 2.2.0 | PyNaCl | 1.3.0 |
pyOpenSSL | 18.0.0 | pyparsing | 2.2.0 | PySocks | 1.6.8 |
Python | 2.7.15 | python-dateutil | 2.7.3 | pytz | 2018.4 |
PyYAML | 3.12 | pyzmq | 17.0.0 | requests | 2.18.4 |
s3transfer | 0.1.13 | scandir | 1.7 | scikit-learn | 0.19.1 |
scipy | 1.1.0 | seaborn | 0.8.1 | setuptools | 39.1.0 |
simplegeneric | 0.8.1 | singledispatch | 3.4.0.3 | six | 1.11.0 |
statsmodels | 0.9.0 | subprocess32 | 3.5.3 | tensorboard | 1.12.2 |
tensorboardX | 1.4 | tensorflow | 1.12.0 | termcolor | 1.1.0 |
testpath | 0.3.1 | torch | 0.4.1 | torchvision | 0.2.1 |
tornado | 5.0.2 | traceback2 | 1.4.0 | traitlets | 4.3.2 |
unittest2 | 1.1.0 | urllib3 | 1.22 | virtualenv | 16.0.0 |
wcwidth | 0.1.7 | webencodings | 0.5.1 | Werkzeug | 0.14.1 |
wheel | 0.31.1 | wrapt | 1.10.11 | wsgiref | 0.1.2 |
In addition, the following Spark packages include Python modules:
Spark Package | Python Module | Version |
---|---|---|
graphframes | graphframes | 0.7.0-db1-spark2.4 |
spark-deep-learning | sparkdl | 1.5.0-db1-spark2.4 |
tensorframes | tensorframes | 0.6.0-s_2.11 |
R libraries
The R libraries are identical to the R Libraries in Databricks Runtime 5.2.
Java and Scala libraries (Scala 2.11 cluster)
In addition to Java and Scala libraries in Databricks Runtime 5.2, Databricks Runtime 5.2 ML contains the following JARs:
Group ID | Artifact ID | Version |
---|---|---|
com.databricks | spark-deep-learning | 1.5.0-db1-spark2.4 |
com.typesafe.akka | akka-actor_2.11 | 2.3.11 |
ml.combust.mleap | mleap-databricks-runtime_2.11 | 0.13.0 |
ml.dmlc | xgboost4j | 0.81 |
ml.dmlc | xgboost4j-spark | 0.81 |
org.graphframes | graphframes_2.11 | 0.7.0-db1-spark2.4 |
org.tensorflow | libtensorflow | 1.12.0 |
org.tensorflow | libtensorflow_jni | 1.12.0 |
org.tensorflow | spark-tensorflow-connector_2.11 | 1.12.0 |
org.tensorflow | tensorflow | 1.12.0 |
org.tensorframes | tensorframes | 0.6.0-s_2.11 |
Versatil Markdown is a hypernotebook built around Markdown/CommonMark, with 1Password integration, HTML/CSS support, syntax highlighting, frictionless keyboard flow, smart drag-n-drop insertion, multiple themes, document aliasing, and more.
Versatil Markdown 2 0 50 Ml Volumetric
Compatibility: macOS 10.12 or later 64-bitHomepage: https://versatilapp.com
https://rapidgator.net/file/2f55f635371fbc8bc06400af412868e6/ase8l.Versatil.Markdown.2.1.0.rar.html
nitroflare_com:
https://nitroflare.com/view/7E9573D2D3B7A77/ase8l.Versatil.Markdown.2.1.0.rar
uploadgig_com:
https://uploadgig.com/file/download/6C0b1e90D6d5E5bf/ase8l.Versatil.Markdown.2.1.0.rar